Introduction
Telegram hosts thousands of groups and channels associated with extremism. This Insight contributes to a growing literature applying computational social science methods to understand extremist use of the Internet. I used the example of Telegram channels associated with the Russian Imperial Movement (RIM) to illustrate how online discourse communities—where discourse functions through networks to further shared objectives—might be mapped using topic modelling and network analysis. This exploratory methodology does not depend on narrative content particular to RIM or on the unique technical features of Telegram, therefore, it has the potential to map extremist discourse communities on other social media sites such as Facebook and Instagram.
This Insight is drawn from the paper ‘Mapping Extremist Discourse Communities on Telegram: The Case of the Russian Imperial Movement and Its Affiliates’. Associated datasets, code, and supplementary materials are available on GitHub.
The Russian Imperial Movement
In 2020, the Russian Imperial Movement (RIM) became the first white supremacist group labelled ‘Specially Designated Global Terrorists’ by the US State Department. Belew and Gutierrez argue that the broader white power movement is more organised and cohesive than is commonly understood, as well as being “profoundly transnational.” RIM has international connections with other extremists and may have sent fighters to Syria, Ukraine, and Libya. They have also provided paramilitary training to Russian citizens joining their affiliate group, Imperial Legion, in Ukraine and to foreigners responsible for bomb plots in their home countries. I selected RIM for this analysis due to their prominence among white supremacist groups, the threat their transnational connections present to international security, and the relative accessibility of their associated channels on Telegram.
RIM has been characterised as aligning with ‘Siege culture,’ a strain of neo-Nazi ideology. Siege culture is defined by the themes of antisemitism, racism, homophobia, anti-abortion, chauvinism, sexual violence, anti-law enforcement and anti-government sentiments. Its adherents advocate for violence and societal systemic collapse. Further work has found that themes in RIM ideology also include religion, a historical vision of imperial Russia, ethnicity, the concept of ‘Western’ culture or a Western world, migration, and Russian domestic politics.
Methodology
To collect Telegram message data for RIM and its affiliates, I began with the channel Русское Имперское Движение (Russian Imperial Movement), which had 2,160 subscribers at the time. I reviewed their messages and identified the 24 most recent channels they had forwarded messages from, referred to here as affiliate channels. I consider these channels ‘public’ spaces due to their large number of subscribers (ranging from 345 to 1,318,490). I then used the 4Cat Capture and Analysis Toolkit (4CAT) to retrieve messages from RIM and affiliate channels that were posted between 1 January and 15 October 2022, resulting in a dataset of 56,866 messages. After filtering this dataset to contain only messages with ‘t.me/*’ (the beginning characters of Telegram channel or message URLs), I obtained 2,731 messages from 19 channels.
Using the ‘URL co-occurrence network’ feature in 4CAT and the network analysis and visualisation software Gephi, I created a co-occurrence network of all Telegram URLs that were included in the same message. The final co-occurrence network includes 725 nodes (Telegram URLs) and 5,473 edges (co-occurrences of URLs in the same message). It is drawn from approximately 52 messages from 13 channels.
Topic Modeling
Latent Dirichlet Allocation (LDA), a popular topic modelling algorithm, has been applied to social media data to study diverse issues such as health, environmental policy, and protests. This algorithm identifies the probability of a word appearing in a topic and a topic appearing in a document. The number of topics is determined by the researcher, and the probability values are calculated through an iterative process based on word co-occurrence patterns.
To apply LDA, I created 725 documents, each representing a Telegram URL in the network. I then added text to each document if it contained the corresponding URL. This step in the analysis removed any messages without Telegram URLs, resulting in 1,540 messages being used in the topic model. I set the number of topics at 10—Table 1 displays the top ten most relevant words associated with each topic in the model, with translations provided in parentheses.
Topic | Label | Keywords | |||||||||
---|---|---|---|---|---|---|---|---|---|---|---|
0 | Russia and immigration | каналы (channels), вообще (in general or generally), эксперта (expert), эксперты (experts), беженцев (refugees), решил (decided to), телеграм (Telegram), россию (Russia), кстати (by the way or incidentally), рф (Russian Federation), сша (USA), dimsmirnov175, verysexydasha, joinchat, удаленка (work from home) | |||||||||
1 | History | города (city), история (history or story), пошло (commonly, commonplace, or went), москва (Moscow), московского (Moscow), истории (history or story), полка (regiment), факты (facts), москве (Moscow), метро (metro), каком (which), правда (true or truth), китай (China or Chinese), канал (channel), moshistory | |||||||||
2 | Russia- Ukraine relations | русские (Russian), играть (play), русофобов (Russophobes), стоит (cost or worth), поэтому (therefore), украину (Ukraine), причём (moreover), сбор (collection or tax), русофобия (Russophobia), проблема (problems), учить (to teach, learn, or punish), средств (funds, means, or resources), tsarkrest, россии (Russia), русских (Russians) | |||||||||
3 | Russia- Ukraine War | движение (movement), русских (Russians), более (more), украине (Ukraine), россия (Russia), больше (more), notes_veterans, людей (people), украины (Ukraine or Ukrainian), всу (Armed Forces of Ukraine), наших (our), россии (Russia), nbondarik, русские (Russian), канал (channel) | |||||||||
4 | War (general) | aviatica, impnavigator, вестник (newspaper or journal), оперативная (operational), войны (war or warfare), militarymaps, морские (sea, marine, or maritime), ядерный (nuclear), линия (line), контроль (control), zvezdanews, ru, пво (air defense), морской (sea, marine, or maritime), новости (news) | |||||||||
5 | History | фактов (facts), прошу (please, ask, or beg), граждан (citizens or nationals), москве (Moscow), prezidentgordonteam, месте (place or area), рф (Russian Federation), москвы (Moscow), история (history or story), истории (history or story), текст (text) , фронт (front or front line), ответственности (responsibility or liability), com, moshistory | |||||||||
6 | Russia and immigration | com, средней (average), жители (people or residents), фронте (front), чат (chat), решение (decision), ответственности (responsibility), мигрантов (migrants), привлечь (attract, draw, or bring), территории (territory), статье (article), vk, города (city), ук (criminal code), рф (Russian Federation) | |||||||||
7 | Thanking | благодарим (thank), подводных (underwater, undersea, or submarine), subforcherald, prbezposhady, новостей (news), улыбаемся (smile), машем (wave or waving), бизнес (business), tolk_tolk, поздравляем (congratulations), planeradar, zangaro, korea, читателей (readers), bbbreaking | |||||||||
8 | Lawmaking | mariashukshina, кавказ (Caucasus), депутат (deputy, member, or lawmaker), аудиторию (audience), канал (channel), obrazbuduschego2, russica2, проект (project), граждан (citizens or nationals), людей (people), kremlebezbashennik, bigtransfer2024, дмитрий (Dmitry), народ (people or nation), страны (country) | |||||||||
9 | Religion and tradition | жизни (life or living), правильно (right or correct), бог (God), традиции (tradition, traditions, or traditional), интернет (internet), юмором (humor), imsindi_z, наших (our), сергея (Sergei), россии (Russia), записки (note or brief), anti_rubra, целом (general or whole), expensive_hurma, vladlentatarsky |
Table 1: Most Relevant Words for Each Topic
The topic modelling results largely align with prior qualitative findings on RIM ideology. Topics reflect themes pertaining to history (topics 1 and 5), Russia-Ukraine relations (topic 2), the Russia-Ukraine war (topic 3), war in general (topic 4), Russia and immigration (topics 0 and 6), religion and tradition (topic 9), and lawmaking (topic 8). Topic 7, which appears to represent thanking readers of a channel, is likely specific to this online context.
I assigned the primary topic for each Telegram URL in the network based on the most prominent topic for its document. Figure 1 shows the co-occurrence network coloured by URL topic. This network graph illustrates that the topics of war, the Russia-Ukraine war, and thanking are potentially associated. The lawmaking topic also has a distinct cluster. Other topics appear to be distributed in smaller clusters throughout the network.
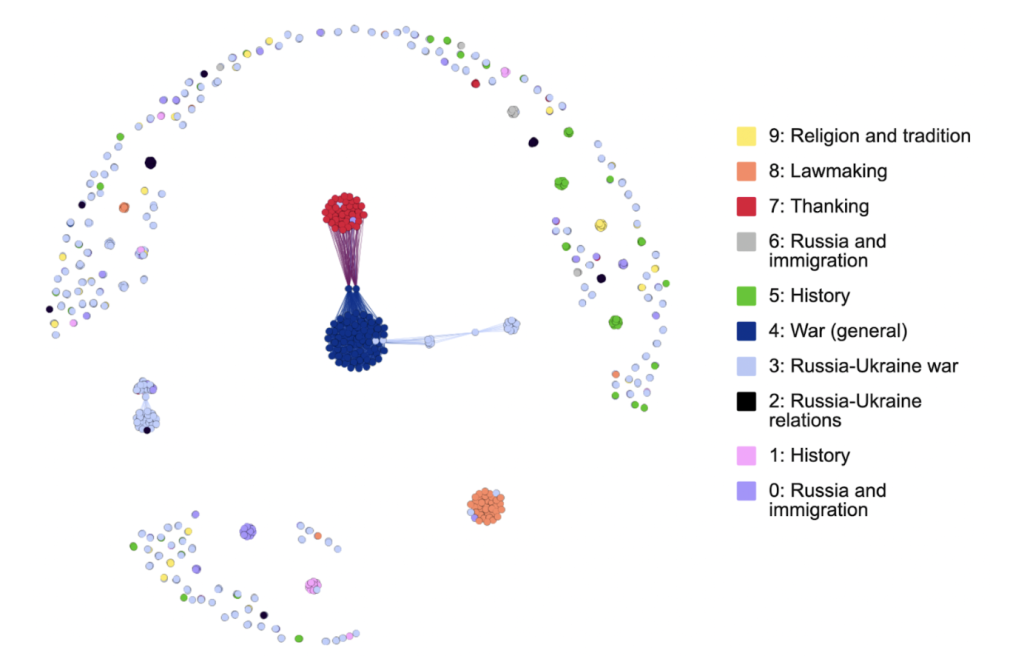
Fig. 1: Telegram URL Co-Occurrence Network Coloured by Topic
Network Analysis
Two of the major approaches to detecting communities in networks are the Louvain algorithm, which finds communities based on the density of connections, and the Girvan-Newman algorithm, which identifies community boundaries. I applied both Louvain and Girvan-Newman community detection methods to the Telegram URL co-occurrence network to examine whether the relationships between topics are strong enough to result in communities focused on similar topics, even when using different community detection methods. Figures 2 and 3 show the distribution of topics in each Girvan-Newman and Louvain community, respectively.
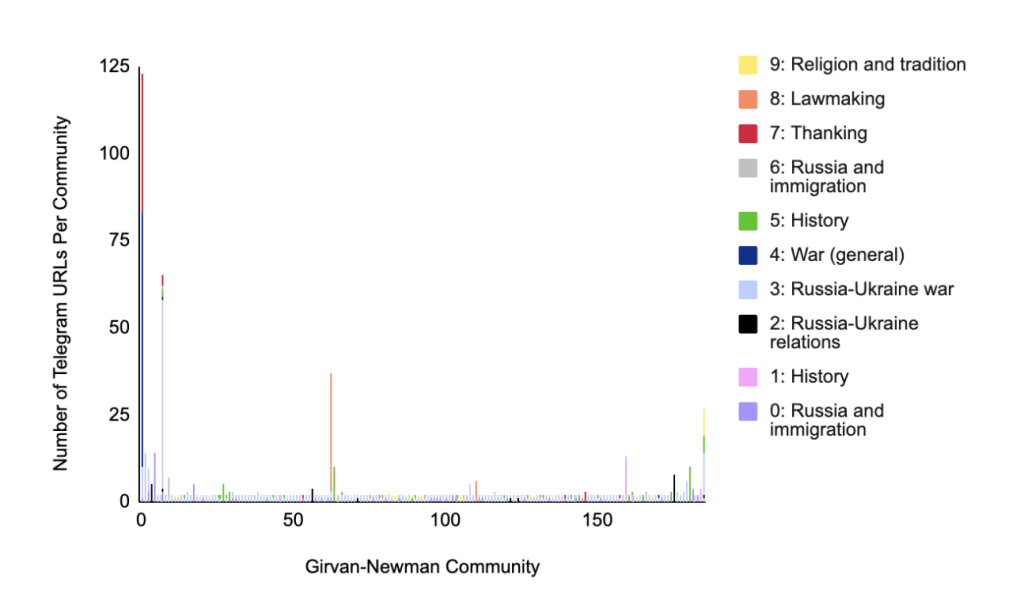
Fig. 2: Topic Distribution by Girvan-Newman Community
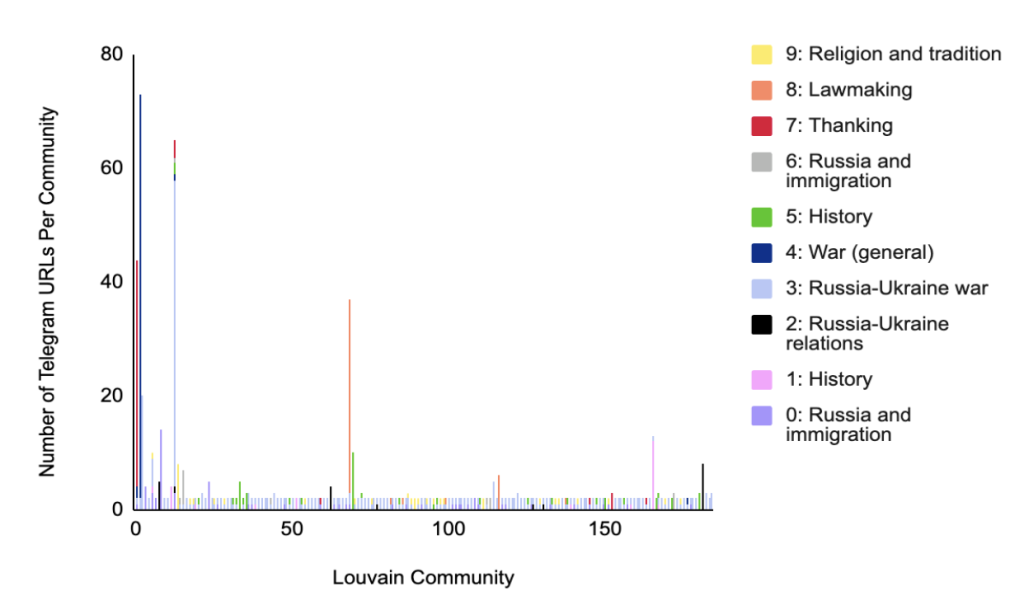
Fig. 3: Topic Distribution by Louvain Community
These results show that the relationships between topics may be robust to different detection methods, as the larger Louvain and Girvan-Newman communities tend to be characterised by the same topics. However, the largest Girvan-Newman community is characterised by two topics (war and thanking) and the largest Louvain community is characterised by one (war); this difference could indicate that the apparent associations between topics are influenced by which detection method is applied. Therefore, the comparison of communities identified through each method is inconclusive.
Conclusion
This research indicates that it may be possible to map discourse communities created by the Russian Imperial Movement (RIM) and its affiliates on Telegram through topic modelling and network analysis. This can be seen in Fig. 1, as the major clusters of co-occurring Telegram URLs tend to be devoted to a primary topic. I argue this network feature can be interpreted as a discourse community.
The example of discourse communities created by RIM and its affiliates on Telegram shows the utility of network analysis and topic modelling for conducting an initial exploratory analysis of how an extremist group operates online. These methods could potentially be applied to other social media platforms such as Facebook and Instagram that allow users to post URLs pointing to groups in messages or posts, and allow researchers to collect this text. However, the more robust moderation of extremist rhetoric on these platforms may present challenges for research. Content moderation may lead movements and groups to use multiple platforms to form discourse communities, which may make it more difficult for researchers to identify accounts or groups associated with extremism as groups adapt to avoid moderation.
Directions for future work include investigating how these discourse communities change over time and in response to relevant events, such as the February 2022 escalation in the Russia-Ukraine war, by analysing how the network and topics discussed change over time. This type of analysis could enable the study of causal questions, such as examining the impact of external events and pressures on extremist groups and how those groups respond on- and offline. Another potential line of future research could be how groups respond to the introduction of various forms of true and false information, providing insight into the relationship between mis- and disinformation and extremist group behaviour.
Sara Morrell is a Ph.D. student at Northeastern University studying political science. Her research focuses on online extremism and international law in cyberspace.